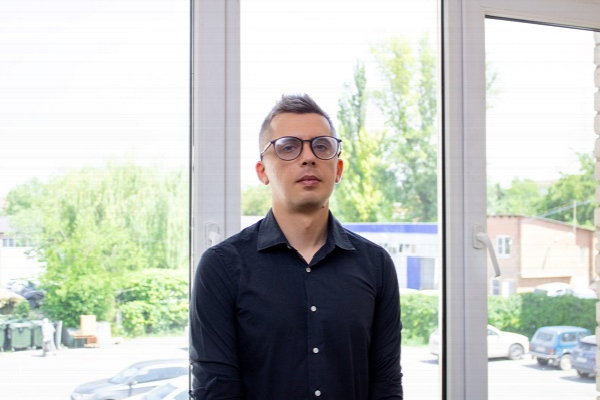
A study conducted by scientists from Southern Federal University and St. Petersburg State Maritime Technical University demonstrates the revolutionary capabilities of artificial intelligence in predicting long-term climate change.
Meteorology and climatology are sciences in which the accuracy of forecasts can save lives and prevent economic losses. The world's media often manipulate facts by passing off one or another mathematical model as a real forecast of climate change. So, back in the 1960s, American researchers convincingly proved that by the year 2000 the temperature across the Earth would rise by 2.5 degrees, but this never happened.
No computer program could take into account all the climate-forming factors necessary for an accurate forecast. This was before the advent of deep machine learning — artificial intelligence, which, if it gives an incorrect forecast, works on errors.
At the Southern Federal University, Denis Krivoguz, a leading researcher at the Department of Oceanology of the Institute of Earth Sciences of the Southern Federal University, is engaged in the use of deep machine learning to study climate change. Denis Krivoguz is a participant in the postdoctoral program of the Southern Federal University within the framework of the Priority 2030 Development Program (national project "Science and Universities").
"Modern challenges in climate forecasting require the use of advanced technologies. Our work is aimed at exploring the potential of deep learning in this area, which can significantly improve the accuracy of forecasts and contribute to the development of new approaches to monitoring and management of climate resources," said Denis Krivoguz.
The architectures of deep recurrent neural networks (DRNN) and DRNN with Gated Recurrent Units (DRNN-GRU), studied in the work "Improving long-term air temperature forecasting using deep learning architecture", have shown the outstanding effectiveness of neural networks in long-term temperature forecasting tasks. The use of such models allows not only to improve the accuracy of forecasts, but also to better understand climate processes, which is especially important in the light of global climate change.
Denis Krivoguz's supervisor, Associate Professor Alexander Ioshpa, stressed that the development of neural networks for climatology is a long—awaited collaboration of mathematical sciences and Earth sciences.
"When an ideal mathematical model appears that takes into account all the factors affecting the climate and proves its effectiveness with an accurate weather forecast for at least six months, it will be a Nobel Prize," Alexander Ioshpa believes.
A neural network can analyze large amounts of data and identify the most significant factors for the weather. For forecasting in the Rostov region, for example, take into account not only the movement of large atmospheric fronts, but also currents in the Black and Azov Seas, soil temperature in neighboring regions, and so on.
"The importance of accurate climate forecasting cannot be overestimated. This is not only a matter of scientific interest, but also a critically important aspect for many sectors of the economy, including agro-climatology and agriculture. In the conditions of the Rostov region, which is one of the leading agricultural regions of Russia, accurate prediction of weather conditions can be of great importance for planning agricultural work, optimizing resources and managing crops," said Denis Krivoguz.
As part of the study, work was carried out with an extensive set of data collected from 1961 to 2023, including from the meteorological station of the Institute of Earth Sciences of the Southern Federal University, operating since 2016. The data included air temperature, atmospheric pressure and precipitation levels, which allowed for a comprehensive analysis of climatic conditions over a long time interval.
Such a difficult task required a large amount of scientific research and experiments, in which the Institute of Robotics and Intelligent Systems (IRIS) of St. Petersburg State Technical University, specializing in the research and creation of intelligent robotic complexes and information processing systems based on artificial intelligence, took an active part
Anton Zhilenkov, Director of IRIS, Dean of the Faculty of Digital Industrial Technologies at St. Petersburg State Technical University, said that one of the main directions being developed at the Institute of Robotics and Intelligent Systems, in addition to robotics, is the creation of information processing systems, predictive systems based on artificial intelligence technologies and machine learning methods.
"In the course of our joint research, we reviewed and compared various architectures of neural networks. Special attention was paid to Deep Recurrent Neural Networks (DRNN) and its variations with Gated Recurrent Units (DRNN-GRU) for their ability to analyze sequential data and identify long-term dependencies in time series. These models demonstrated significant superiority in forecasting accuracy compared to traditional methods, thanks to a deep analysis of time dependencies and high adaptability to changes in data," shared Anton Zhilenkov.
In addition, attention-driven architectures such as LSTM-Attention and RSLSTM-Attention were studied, which allowed models to focus on the most significant aspects of input data while taking into account their context and interrelationship. Despite the fact that these models showed good results in some tasks, in general comparison they were inferior to DRNN and DRNN-GRU in the accuracy of temperature prediction.
The use of DRNN and DRNN-GRU models has made significant progress in the accuracy of forecasts. The analysis of the comparison of predicted and actual temperature data is a key part of the study aimed at evaluating the accuracy and reliability of deep learning models. As part of the work carried out, a detailed comparison of the results obtained using the DRNN and DRNN-GRU models with real meteorological data was performed.
The forecasts generated by the DRNN model in most cases demonstrated a high degree of compliance with the actual temperature indicators with small deviations within ±2 °C. This indicates the effectiveness of the model in predicting temperature, taking into account long-term time series and variability of climatic conditions.
For example, when analyzing temperature data for the summer period of 2023, DRNN forecasts showed a deviation from the actual data by 1.5°C, which is an acceptable indicator for long-term forecasting. The DRNN-GRU model, in turn, demonstrated even more accurate results, reducing the average deviation to ±1.2°C. This was made possible by the use of GRU blocks, which allow for more efficient processing and memorization of information about previous system states, which is critically important for taking into account long-term patterns in the data.
"It is worth noting that when comparing forecasts with actual data during periods of extreme weather conditions, such as unusually warm winters or sharp cold spells in summer, there was an increase in deviations. Despite this, both models successfully predicted general temperature trends, demonstrating their suitability for practical use in long—term weather forecasting systems," explained Denis Krivoguz.
Climate change, which affects the frequency and intensity of droughts, floods and other extreme weather events, directly affects agricultural production. Therefore, the development and implementation of advanced forecasting methods are becoming key to adapting to these changes. Artificial intelligence and deep learning open up new perspectives in this field, allowing you to predict temperature conditions with great accuracy for a long period.
"Our research opens up new opportunities for using deep learning in meteorology and climatology. We hope that this area will actively develop, making a significant contribution to the fight against the negative effects of climate change and helping society adapt to new conditions," Denis Krivoguz summed up.
The developed models demonstrating high forecasting accuracy can become the basis for the creation of new management systems in agriculture that can adapt to changing climatic conditions. This opens the way for optimizing the processes of sowing, watering, fertilizing and harvesting, increasing the efficiency of using land and water resources, which in turn can contribute to increasing productivity and sustainability of the agricultural sector of the Rostov region and the whole of Russia.
Short link to this page sfedu.ru/news/75800